Biomni
A Generalist Biomedical AI Agent





Authors
Kexin Huang1,⇤,‡, Serena Zhang1,⇤, Hanchen Wang1,2,⇤, Yuanhao Qu3,4,5,⇤, Yingzhou Lu5,⇤, Yusuf Roohani1,6, Ryan Li1, Jingtian Zhou6, Junze Zhang3,5, Di Yin3,5, Shruti Marwaha6, Jennefer N. Carter6, Xin Zhou5, Peng He8, Matthew Wheeler6, Jonathan A. Bernstein6, Michael Snyder5, Le Cong3,5, Aviv Regev2, and Jure Leskovec1,‡
1Department of Computer Science, Stanford University School of Engineering, Stanford, CA, USA
2Research and Early Development, Genentech, South San Francisco, CA, USA
3Department of Pathology, Stanford University School of Medicine, Stanford, CA, USA
4Cancer Biology Program, Stanford University School of Medicine, Stanford, CA, USA
5Department of Genetics, Stanford University School of Medicine, Stanford, CA, USA
6Arc Institute, Palo Alto, CA, USA
7Department of Cardiovascular Medicine, Stanford University School of Medicine, Stanford, CA, USA
8Department of Pathology, University of California, San Francisco, San Francisco, CA, USA
9Department of Pediatrics, Stanford University School of Medicine, Stanford, CA, USA
⇤Equal contribution.
‡Corresponding authors. Email: kexinh@cs.stanford.edu, jure@cs.stanford.edu
Abstract
Biomedical research underpins progress in drug discovery, clinical care, and our understanding of human health and disease. However, biomedical research is increasingly constrained by repetitive and fragmented workflows that slow discovery, limit innovation, and underscore the need for a fundamentally new way to scale scientific expertise. Here, we introduce Biomni, a generalist biomedical AI agent designed to autonomously execute a wide spectrum of research tasks across diverse biomedical subfields. To systematically map the biomedical action space, Biomni first employs an action discovery agent to create the first unified agentic environment—mining essential tools, databases, and protocols from tens of thousands of publications across 25 biomedical domains. Built on this foundation, Biomni features a generalist agentic architecture that integrates large language model (LLM) reasoning with retrieval-augmented planning and code-based execution, enabling it to dynamically compose and carry out complex biomedical workflows—entirely without relying on predefined templates or rigid task flows. Systematic benchmarking demonstrates that Biomni achieves strong generalization across heterogeneous biomedical tasks—including genetic variant prioritization, drug repurposing, rare disease diagnosis, microbiome analysis, and molecular cloning—without any task-specific prompt tuning. Real-world case studies further showcase Biomni's ability to interpret complex, multi-modal biomedical datasets and autonomously generate experimentally testable protocols. Biomni envisions a future where virtual AI biologists operate alongside human scientists to dramatically enhance research productivity, clinical insight, and personalized healthcare.
Key Features
Explore the architecture and capabilities of Biomni
1. First Biomedical Agentic Environment with Unified Action Space

Biomni integrates tools, databases, and software across multiple biomedical subfields including genomics, genetics, molecular biology, cell biology, physiology, biochemistry, microbiology, pharmacology, bioengineering, biophysics, and pathology.
2. Superior Zero-shot Generalization

Biomni demonstrates robust zero-shot performance across eight previously unseen, real-world biomedical scenarios spanning multiple biomedical subfields, without any task-specific fine-tuning or prompt engineering.
3. Complex Multi-modal Data Analysis

Biomni analyzes 458 wearable sensor files to generate physiological hypotheses, completing in 35 minutes what would take an expert bioinformatician several weeks of work.
4. Expert-Level Wet-lab Protocol Design

Biomni designs CRISPR cloning protocols in minutes that would take even experienced biologists hours to create, with experimentally validated results.
Key Results
Major findings and contributions of the Biomni research project
Biomni creates the first unified agentic environment by systematically mining essential tools, databases, and protocols from tens of thousands of publications across 25 biomedical domains.
Biomni achieves human expert-level performance on challenging biomedical benchmarks, including database querying (74.4% accuracy) and sequence analysis (81.9% accuracy).
Biomni demonstrates strong zero-shot generalization across eight diverse biomedical tasks, including variant prioritization (+78.9%), rare disease diagnosis (+10.3%), and microbiome analysis (+180.0%).
Biomni autonomously analyzes complex multi-modal datasets, including 458 wearable sensor files and single-cell multiomics data, generating novel biological insights and hypotheses.
Biomni designs molecular biology experiments with expert-level accuracy, demonstrated through successful implementation of CRISPR guide RNA cloning experiments.
Biomni envisions a future where virtual AI biologists operate alongside human scientists to dramatically enhance research productivity, clinical insight, and personalized healthcare.
See Biomni in Action
Explore real-world demonstrations of our biomedical AI agent across various applications.
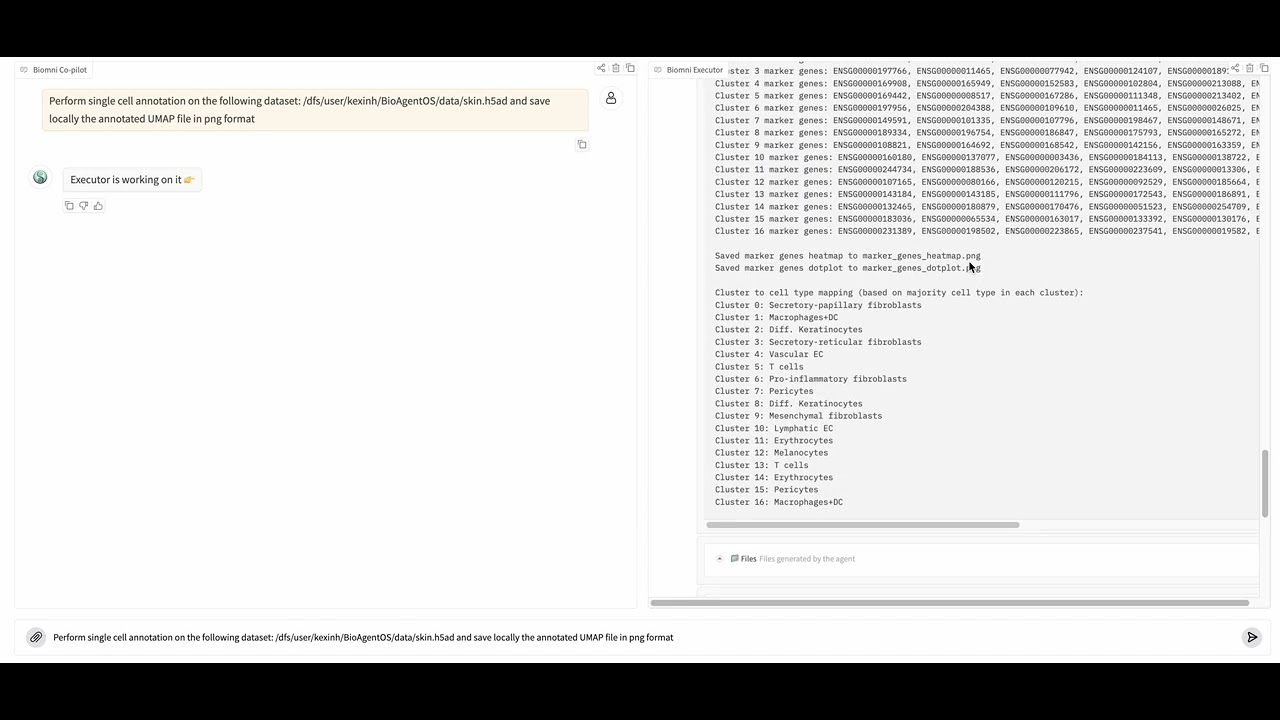
scRNA Cell Annotation
Watch Biomni automatically annotate single-cell RNA sequencing data with high accuracy.
Watch Demo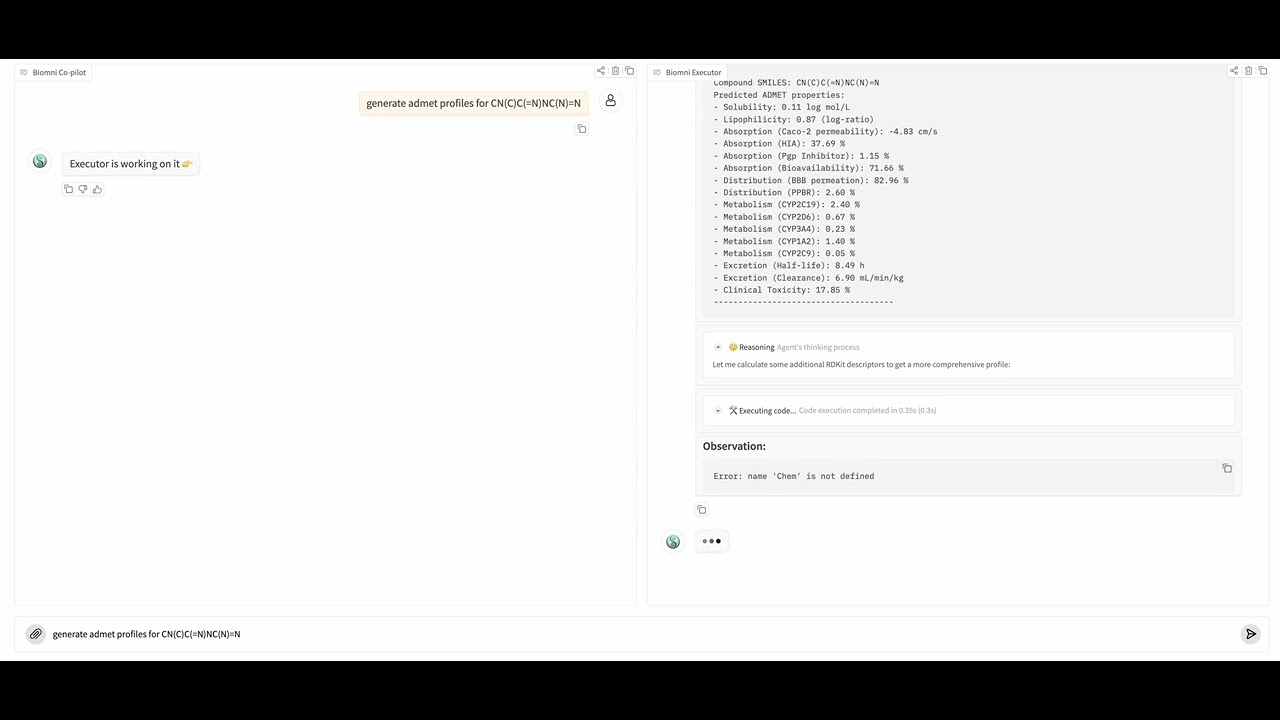
ADMET Property Prediction
See how Biomni predicts absorption, distribution, metabolism, excretion, and toxicity properties of drug candidates.
Watch Demo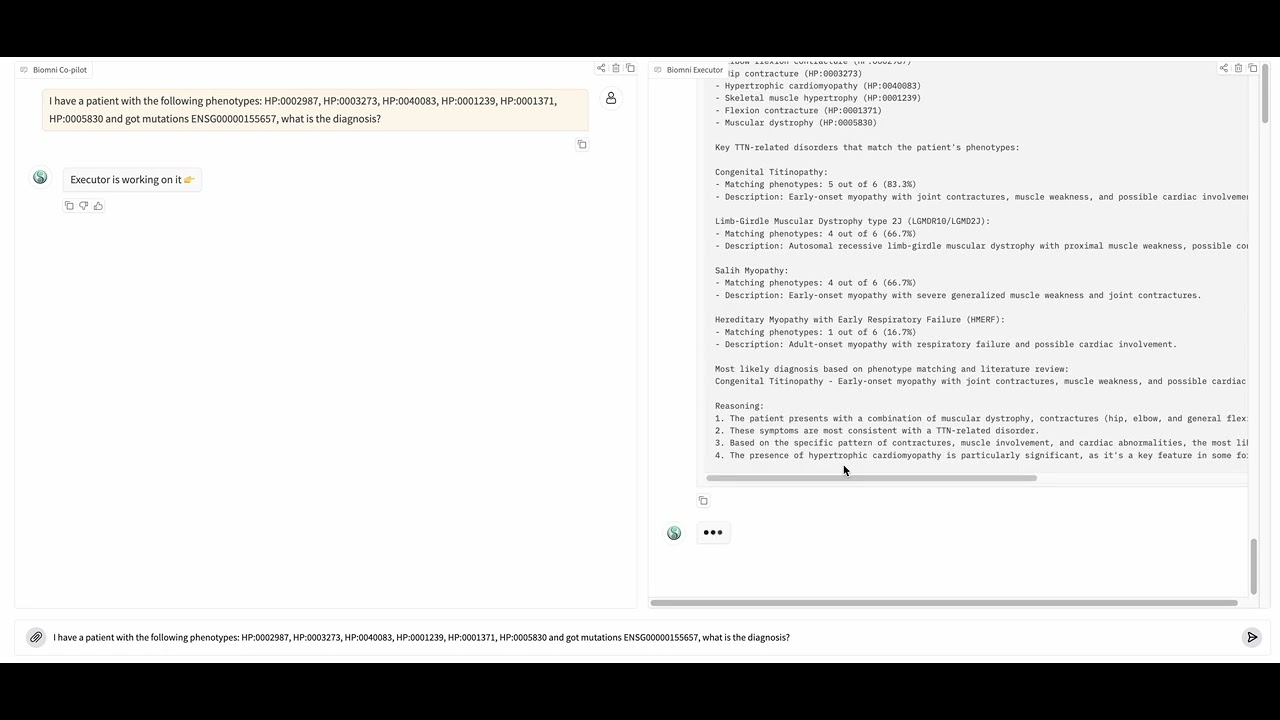
Rare Disease Diagnosis
Watch Biomni analyze patient data to assist in the diagnosis of rare genetic disorders.
Watch Demo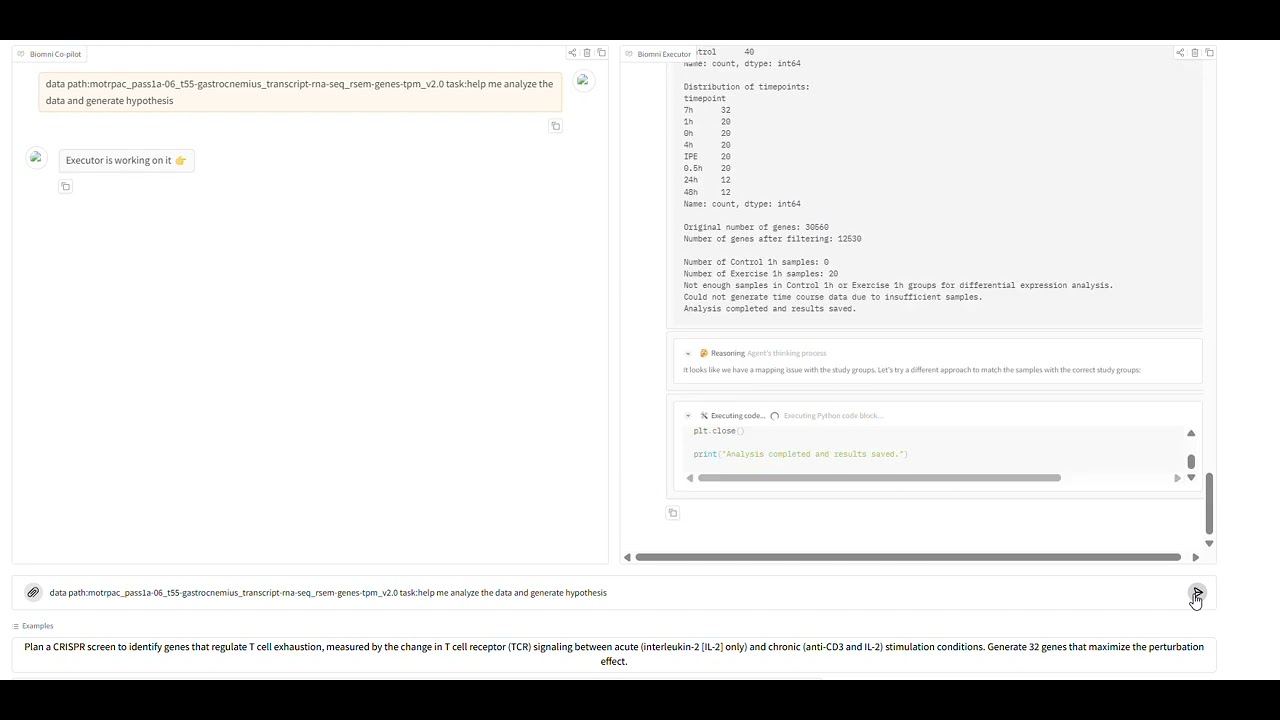
MoTrPac Analysis
See Biomni analyze complex multi-omics data from the Molecular Transducers of Physical Activity Consortium.
Watch Demo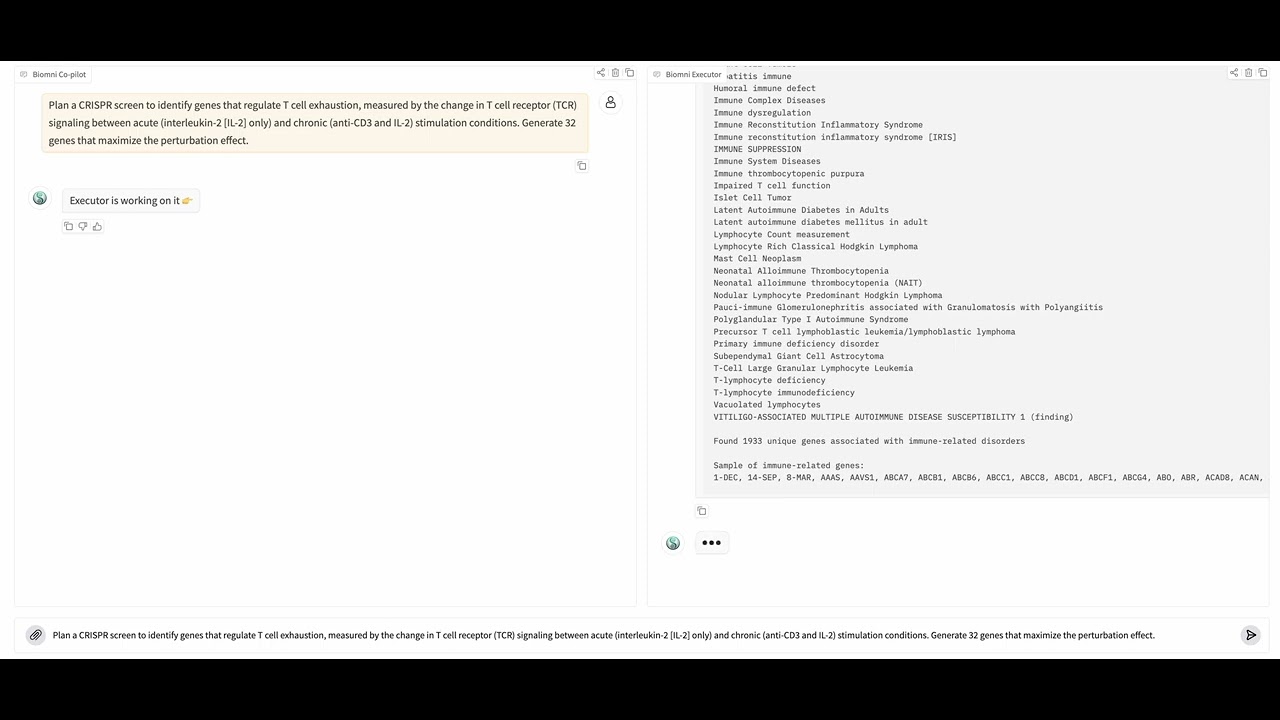
Perturbation Panel Design
Watch Biomni design optimal genetic perturbation panels for functional genomics experiments.
Watch Demo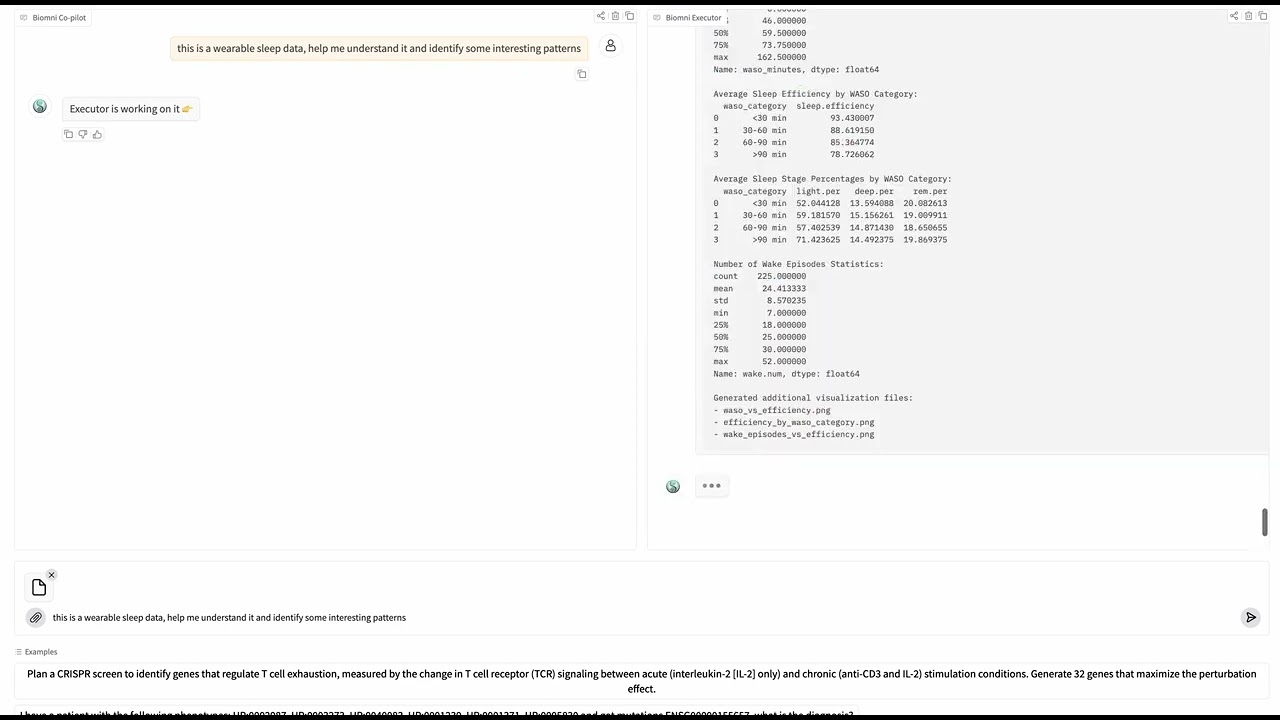
Wearable Data Analysis
See how Biomni analyzes complex wearable sensor data to generate physiological insights.
Watch Demo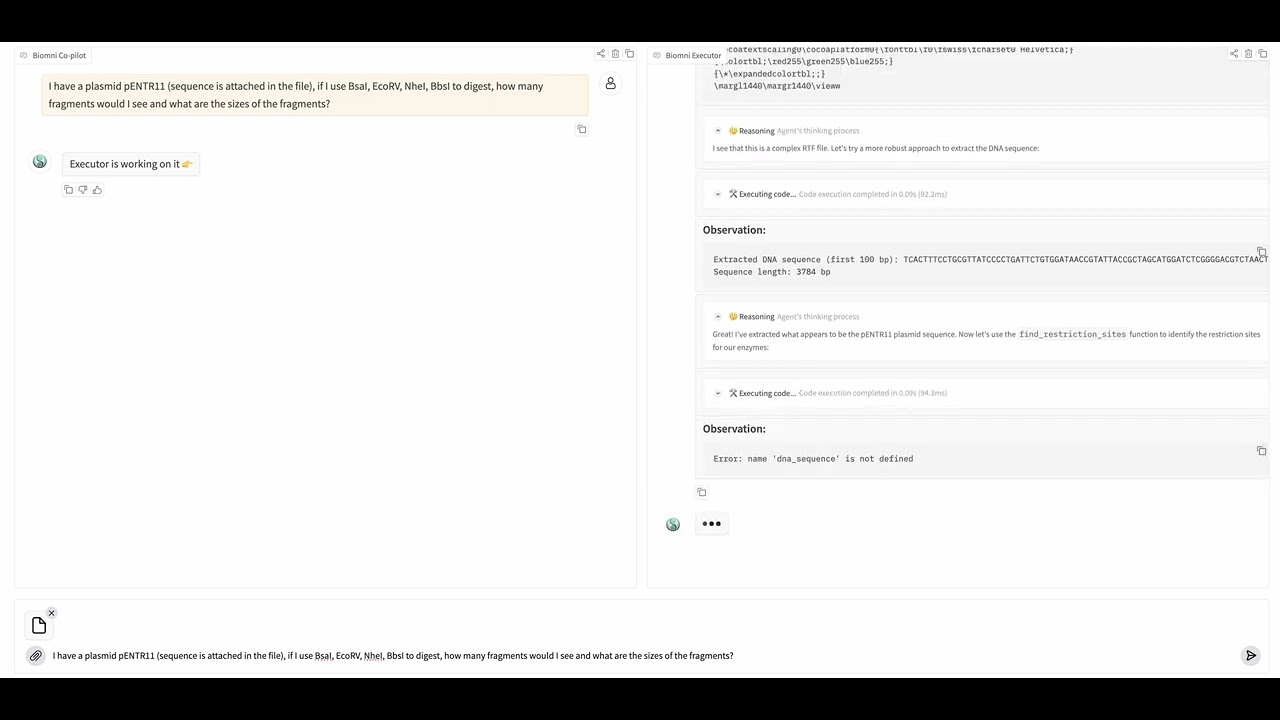
Cloning Design
Watch Biomni design optimal molecular cloning strategies for genetic engineering experiments.
Watch Demo